Quercus Technologies: License Plate Recognition: Past, Present and (a Great) Future
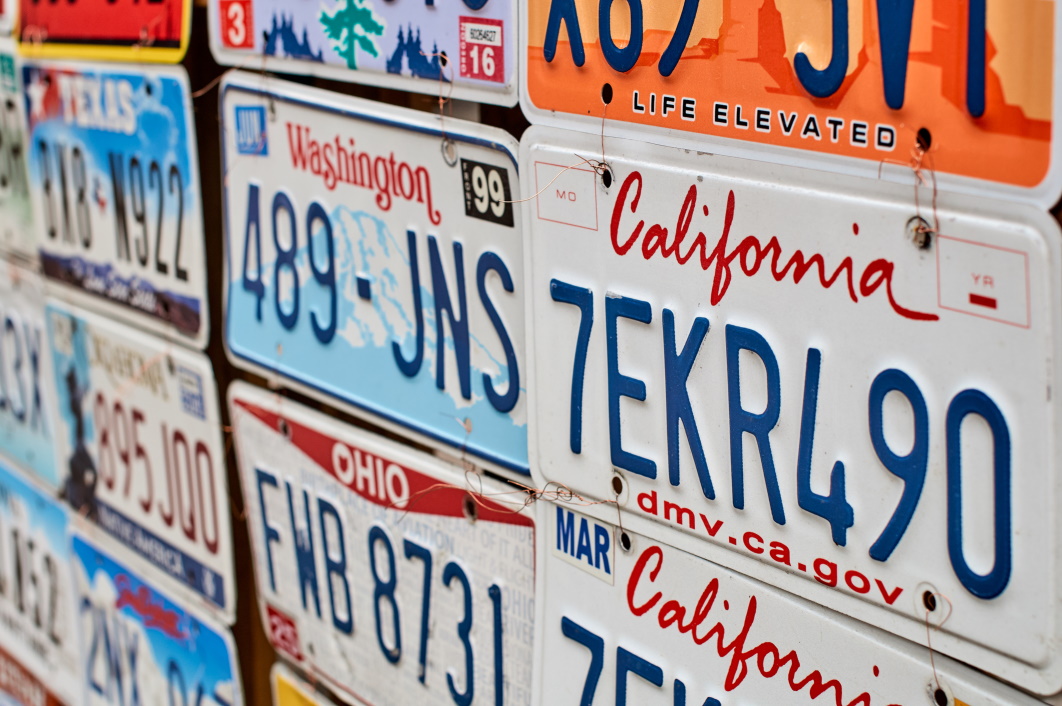
License Plate Recognition (LPR) is a technology that takes a vehicle’s license plate information from an image or group of images. In recent years, LPR, a field of Computer Vision (CV), has been widely used in various sectors related with vehicle monitoring: road safety, parking facility control or automatic toll and parking payment, among many others.
How and When Did the Need to Recognize License Plates Come About?
The first references to an LPR system date back to 1976 when England’s “Police Scientific Development Branch” developed the first system, deployed in 1979. In addition to a technical challenge, which is often the case in research projects, the aim of this application was clear from the outset: increase safety. Since then, the technology has evolved a great deal in its technical aspects as well as its fields of application.
How Has License Plate Recognition Technology Evolved over Time?
At first, these systems were made up by two clearly distinct parts: the first was made up of the optics, with cameras optimized for high-quality image capture. the second was an external CPU with enough computing power to process Optical Character Recognition, or OCR, within a suitable amount of time. Although this initial configuration would still be valid, the evolution of CPU’s toward smaller, more powerful and economical components led systems to evolve toward All-in-One devices, which reinforced their versatility.
It is a well-known fact that Artificial Intelligence has revolutionized the field of programming in recent years. Although AI may sound like a novel concept, the first references to it are from the 1950’s. In 1956, during the Dartmouth conference, a small group of professionals from different fields met and focused their attention on the incipient world of computers, and their potential capacity to show intelligent behavior.
Much progress has been made in this field since then. Over the past decade, still newer technology— Deep Learning (DL)—has sped the development of AI. This methodology is a branch of Machine Learning that is based on learning by example. A model is created that can evaluate examples, in addition to a small set of instructions to modify the model when errors occur. The aim is for the system to autonomously learn from its own experience and, during the training and learning process, be able to identify patterns that allow it to solve a complex problem in a highly accurate way. One of the most common techniques for the implementation of DL is based on the use of neural networks.
What Are Neural Networks, and How Do They Work?
The artificial neural networks model is inspired by the way the human brain works. They are made up of a collection of nodes called artificial neurons, which are grouped in layers, making up a neural network. The nodes are interconnected so that a signal at the input can travel until it generates an output. Each neuron has a weight, a numerical value that modifies the input received. The new values generated leave the neurons, and continue traveling through the network, reaching an output that is the network’s calculated prediction. The more layers it has, the more complex the network, and likewise, the more complex the problems it will be able to solve.
This model is meant to be capable of adjusting itself so it can perform complex tasks that cannot be programmed through classical rule-based programming. This procedure takes place during the training process, in which data are entered as input. Depending on the result reached, the weights of the neurons are adjusted according to the error rate produced. This method is known as “backpropagation”. “Backprop”, or BP, enables the network to learn automatically until the desired results are achieved, making them highly accurate.
Deep Learning Applied to License Plate Recognition Technology
Those who are devoted to research and development in the field of license plate recognition face a number of challenges that must be overcome for the recognition process to be effective. First, the system has to be fast and effective under different image capture conditions, such as lighting (day or night), position and camera specifications (angle, perspective, distance, resolution). On another note, the broad variety of license plate types that feature different colors, typographies, and alphabets make license plate recognition a complex and stimulating technical challenge.
Deep learning is growing fast thanks to its application in Big Data and the IoT. This evolutionary progress is also being brought to bear in the world of LPR, for the industry to keep doing what it has always worked for: improve by seeking new of horizons of perfection. It must be borne in mind that classic CV techniques have reached their technical limits. They are no longer state-of-the-art technology, at least in non-controlled scenarios, as it is impossible to program rules that can handle the infinite combinations of input data that exist in the real world.
Therefore, if every stage of the license plate recognition process is made into an optimization problem with complex error distribution, we will get the most out of DL, and reach very high accuracy rates. But this requires amounts of memory and processing time that differ from those used up to now. To meet the goal of getting efficient response times in more or less complex models, special processing units such as Graphics Processing Units, (GPU’s) or Neural Processing Units (NPU’s) are used.
History Repeats Itself
Today, we are at a turning point similar to that of CPU’s several years ago: this technology is still not cheap enough to use it on a broad scale. Basically, we face two possibilities: embrace the challenge of an integrated All-in-One system, in which the evolution of the hardware has a (relatively) long way to go, or break away from the All-in-One philosophy, and perform the DL processing piece in an external server (or the cloud). Technology evolves quickly. Something that has a prohibitive cost today may be absolutely affordable in a matter of months. The future holds infinite possibilities, and all of them improve the quality and general effectiveness of our license plate recognition (LPR) systems.
A Promising Future
Summing up, LPR technology is anything but stagnant. The appearance of DL has brought about new momentum that is empowering us to reach extremely high success rates and resolve complex situations much more agilely than ever before. Now is the time to keep investing in research of this field. At Quercus Technologies, we aim to continue on the path of providing our customers with the best parking solutions, and helping them overcome any challenges they face.
Article Authored By
Úrsula González-Nicolás - R&D Computer Vision Team responsible
About Quercus Technologies
Quercus Technologies offers optimal solutions for any type of parking facility, maximizing efficiency in its management, and improving the customer's parking experience. The company develops and manufactures highly technological products that aim to improve mobility and security in parking facilities and it is known worldwide for its highly reliable license plate recognition (LPR) technology. Quercus' solutions include All-in-one LPR units, parking guidance, and LPR-based spot monitoring units, light per space systems, LED displays, kiosks, and the innovative software parking suite, BirdWatch, that relies on all these smart technologies and provides total control of parking facilities.
Comments
There are no comments yet for this item
Join the discussion